CSE 2024 Fall Departmental Demo Day

We're thrilled
to invite you to the thirteenth bi-annual Comp. Sci. & Eng. Fall Demo Day. Student groups from several CSE capstone classes will be presenting the culmination of 3-months of effort, hard work, (metaphorical) blood, sweat (well... caffeine really), and tears (see above).
Davis Hall; 1st and 2nd Floor Atrium
Monday, December 9th, 2024
Schedule
Setup
Setup will start at Noon. Tables will be available for both sponsors (each sponsor will get a table) and demo participants (2 to a table). Easels will be available for participants. If you need power, please let us know! If you have any other special requests, please contact ahunt@buffalo.edu to let me know, and we will do our best to accomodate you. There are two hours reserved for setup - you can come at any time during that period to get organized, but please make sure you leave yourself enough time to be ready to go by 2PM, to give you the chance to network.
Networking
Before we open the atrium to students and the public, we’ll have some time reserved for the participants to come and chat with the sponsors and the judges. Pizza will be there as well (A big thank you to our sponsors!), so that the participants and sponsors can have a chance to eat before demos begin!
Judging
During the demo, judges will circulate to the participants demo stations, and they will be rating each project on a specific set of criteria. Judges, expect to spend approximately five minutes with each team, in order to give you time to see them all. You will be assigned a set of projects to view specifically, but you can feel free to talk to more teams as time permits! Teams, keep this in mind and keep your presentations crisp and to the point!
Prizes
There will be prizes for the top teams selected by the judges. They will be announced in the atrium, and there will be a quick photo op for each winner. Good luck to everyone, and I can’t wait to see you all there!
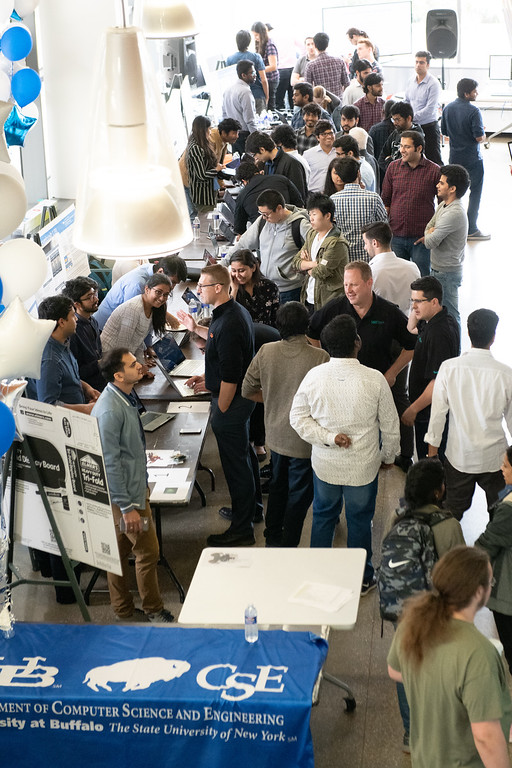
Winners
1st Place - BREATHE
2nd Place - Material Microstructure
3rd Place - The Last Run: Project Hope
Acknowledgements
Judges
- Chris Miller
- Anarghya Das
- Amir Nassereldine
- Jim Brandt
- Karlene Kardysauskas
- Nicholas Myers
- Nick Minor
- Andy Olewnik
- Tyler Poland
- Mary Ruelle
- Kevin Cleary
- Ashton Hannon
- Justin Whitehead
- Hannah Wilcox
- Nicholas MacRae
- Eric Scott
- Hollis Paquette
- Luke Gardner
- Joe Forsyth
- Ethan Blanton
Presented Projects
(CSE662)
- DBSecureGen
-
Graph Db extension to enforce AReBAC priviege
(CSE 410 Game Design)
- Only Up: The Prologue
-
Only Up: The Prologue, draws its inspiration from the highly acclaimed platformer known as Only Up, hailed by many as one of the most challenging games in its class. The original game is no longer available on the Steam marketplace, though it has left a mark on the fans. So we took this as an opportunity to reimagine it and bring it to life with more intense difficulty levels and captivating gameplay that keeps players on their toes. With hope, Only Up: The Prologue will be equally challenging and unforgettable both for advanced players and beginners. Good luck, and have a good climb!
- The Last Run: Project Hope
-
Zombie Parkour game inspired by the Dying Light series you are tasked with delivering the cure to save the human race from the zombie virus.
- Multiverse Run
-
The game is set in a tear in the multiverse. You play as Sonic in the various universes, including his own. Players must navigate each environment, overcoming obstacles to reach the end of the level as quickly as possible.
- Conquest of the Chaos Dragon
-
You play as the evil dragon who has escaped his prison and is trying to destroy the world.
- Area - 51- Z
-
Within the pit of hell that humans once called Earth, players will assume the role of German government official Fredrick Stombon on his hate-filled adventure to send the Z’s back to the fiery cesspit of HELL they sprouted from!
- Resonance
-
A sound-based cave exploration horror game
- Last Stand
-
A round-based third person zombie shooter with advanced gameplay mechanics. Will you survive?
- Search Frenzy
-
Our game concept is based on a mother who is looking for her child. There a three levels the player has to go through to find their child. Theres a list of items to find, once found the next level unlocks.
- Whispers in House of Scares
-
This is a video game created in Unreal Engine 5.The game will be a horror series of escape rooms. Players will have the task of solving numerous puzzles that will require critical thinking and problem-solving skills that will be required to escape.
- Portal 3-ish
-
A video game based off of the Portal franchise, implemented in Unreal Engine 5. Levels are designed by the team members, following the story of the facility's founder being stuck and needed to be freed. As the player, you make your way through the puzzles, going deeper into the chambers, with the ultimate goal of getting to the founder.
- Night Shift At Harper
-
Horror/Story Game Developed in Unreal Engine 5
- Hidden Sands
-
A rouge like game, where you the character need to escape an underground pyramid. Fight enemies, avoid traps, and solve puzzles to get out.
- Brainrot Runner
-
CSE410 Project to learn unreal engine 5. Inspiration taken from subway surfers and other endless runner type games.
- ShotCallers
-
A multiplayer arcade game where 2 players take turns preparing moves and shots that then get released at the same time. Littering the environment with persistent bullet trails that the players have to keep avoiding until the end of the game!
(CSE 302/303)
- EL/R Wireless Localization
-
Indoor Wireless Localization (specifically Wi-Fi) has become quite widespread, there is an active Task Group 802.11bf as well to bring this into standards and there are many learning based solutions to bring localization accuracy to sub-meter level. While, this is great news for indoor automation, there are deployability concerns in terms of generalizability and privacy concerns surrounding it. While, the most popular approach is to use data based ML-models to provide sub-meter accurate localization algorithms, there are still requirements for generalization to different environments and better accuracy. During the first semester, the students will develop a web-app to get data from Wi-Fi routers for a given smartphone. The data is then to be processed online to pass through an ML-model. While, there is a baseline ML-model, they should develop and improve the generalizability and accuracy of the localization algorithm (which would be almost similar to object detection problems). The next stage of the project would be to develop quantifiable differential privacy for these learning models, by adding quantifiable noise to the input images.
- Choreographic Programming
-
Choreographic programming aims to simplify distributed systems / concurrent systems programming by allowing a programmer to specify the system in its entirety - participants, their computations, and communication patterns. From this high level choreographic program, we can synthesize concrete implementations for each node in the distributed system or each thread in the concurrent system.
- Fresumes
-
Fresumes is a platform offering over 500+ free resumes from real people to help employers find talent and job seekers connect with opportunities. With seamless viewing, support for various file formats, and easy access from any device, users can upload or browse resumes effortlessly in just a few clicks.
- Systems Validation Research
-
Our systems validation research, which began with an instructional overview of distributed systems and the investigation of an existing means of evaluating system performance, extended to a central focus on distributed SQL database CockroachDB. Through modifications to the CockroachDB codebase, we evaluated the performance of running YCSB workloads on clusters of CockroachDB nodes with multiple constraints, which include Zipfian constants (the underlying statistical distribution for the workload), read-write fractions (the ratio of YCSB workload operations), and database field lengths. From there, we summarized and analyzed our findings through line graphs to formally represent how various conditions affect the performance of a workload run on a distributed system.
- Find a Mechanic
-
Find a mechanic of FAM for short is a service based webpage and app with the goal of becoming a doordash and/or instacart of trades and services. As of right now we’re focusing on the mechanics trade due to it being the largest and having year round work. This software has 3 core portions. A marketplace webpage for a shop to administer and delegate time, work, and resources internal to customer issues and communicate and do the entire workflow process with customers. The other two portions are an app that has two uses. 1st use is for a worker of the shop to have work delegated to them from the shop webpage that allows them to update on progress of a vehicle and a user facing app that allows them to find a shop of their desired niche by reviews. The user can interact with the shop from start to finish from the app.
- DevU
-
DevU is a modern replacement for AutoLab, designed to provide automated code grading capabilities with extensibility at its core. The platform features a comprehensive API that empowers both instructors and students to develop custom integrations, expanding functionality beyond built-in features.
- Material Microstructure
-
GraSPI is graph-based structure property identifier software implemented as a C/C++ package. GraSPI computes a library of descriptors for a segmented microstructure and computes descriptors that are relevant for organic solar cells performance. The package represents microstructure as a graph and harnesses the graph-based theory to compute wide range of descriptors at low computational cost. A suite of tools for data conversion between various formats and for post-processing the raw results from the graph analysis is provided. Our main objective was to enhance this project by translating it into python using various Python-based libraries.
(Summer2024 Highschool Intern )
- Study of Fake review detection using Machine learning Algorithms
-
Fake review detection has become increasingly im- portant as online platforms rely heavily on user-generated content to influence consumer decisions. Fake reviews can distort public perception, undermine trust in services, and mislead potential buyers. Detecting these deceptive reviews is a challenging task due to their subtle nature and the sheer volume of data on digital platforms. Machine learning (ML) offers a powerful solution to this problem by automatically identifying patterns and anomalies in large datasets that may indicate fraudulent activity. By analyzing textual features and review behavior, ML models can classify reviews as fake or genuine with high accuracy, providing a scalable and efficient way to safeguard the integrity of online platforms. This project implements machine learning algorithms to build a robust system for detecting fake reviews, aiming to enhance trust in digital marketplaces by ensuring the reliability of user feedback
(Independent Study / Research)
- Dementia Voice Dynamics (DVD)
-
Our project, explores the simulation of dementia-like speech patterns using advanced neural networks. By manipulating the weights of Tacotron 2, a popular text-to-speech model, we introduce characteristics such as word repetition, incorrect word usage, and vocal irregularities to mirror symptoms seen in dementia. The generated speech is analyzed through mel-spectrogram comparisons between normal and modified outputs, providing a visual representation of differences in speech patterns. This research aims to enhance understanding of dementia-related vocal cues, with potential applications in both clinical and assistive technologies.
- Efficient Driver Distraction Detection Using HOG Features and Channel Attention Mechanism
-
This project focuses on developing a real-time driver distraction detection model using a lightweight CNN architecture with Histogram of Oriented Gradients (HOG) features and Channel Attention mechanisms. The model accurately identifies driver behaviors with high computational efficiency, making it suitable for deployment in in-vehicle monitoring systems. By providing quick and precise detection of distracted driving, this project aims to enhance road safety and contribute to the development of intelligent transportation systems.
- Harnessing Social Media Sentiments for Strategic Disaster Management
-
In today's digital age, Twitter emerges as a crucial platform for real-time expression and connection, offering a rich dataset for sentiment analysis. This project focuses on harnessing Twitter's rapid data flow to enhance emergency response and humanitarian efforts. Despite challenges like the platform's brief message format and informal language, advancements in machine learning and natural language processing have improved sentiment analysis accuracy. By refining these techniques, the project aims to provide actionable insights for businesses, policymakers, and especially emergency teams, improving societal resilience and response effectiveness during crises. This endeavor not only seeks to elevate public sector responsiveness but also empowers humanitarian agencies with precise, timely data to optimize their emergency interventions.
- WealthWIse
-
Here's a concise, single-paragraph description: WealthWise is a comprehensive financial management platform that revolutionizes how you handle your money by bringing all your bank accounts together in one powerful dashboard. Instead of juggling multiple banking apps and websites, users can seamlessly view, track, and analyze all their financial transactions in a single, secure location. The platform offers real-time balance updates, smart expense categorization, and sophisticated spending analytics that help you understand where your money goes. Whether you're looking to gain better control over your expenses, streamline your financial management, or simply get a clearer picture of your overall financial health.
- Skin Lesion Classification
-
Deep neural networks have demonstrated remarkable success in learning visual representations for image classification across diverse applications. In this study, we introduce a novel neural network architecture tailored for classifying skin lesions based on their visual characteristics and appearance. Our approach utilizes a pretrained ResNet50 backbone, fine-tuned specifically for the task of skin lesion classification using publicly available datasets, including HAM10000 and ISIC. To further enhance classification accuracy, we propose a custom image preprocessing pipeline that highlights critical features while suppressing background noise. Furthermore, we design an innovative loss function that refines the feature space by clustering similar images and distancing dissimilar ones. Our method sets a new benchmark, outperforming existing solutions in the domain of skin lesion classification.
- Adaptive Driver Assistance: Context-based Approach to Pedestrian Safety
-
Pedestrian safety is a key element of Advanced Driver Assistance Systems (ADAS), particularly in urban environments where diverse contextual and behavioral factors influence actions. This project introduces an adaptive driver assistance framework using a single Vision Transformer (ViT) model with Low-Rank Adaptation (LoRA) for efficient multi-task classification, significantly reducing parameters while maintaining high accuracy. Leveraging the JAAD dataset, our approach integrates YOLOv8 for pedestrian detection and a contextual classification framework to assess pedestrian density, road type, time of day, and weather. Pedestrian behavior is modeled by predicting crossing intent, occlusion, and gaze direction, with a rule-based system for adaptive decision-making in critical scenarios. By experimenting with various LoRA techniques—such as AdaLoRA, IA3, LoHA, and LoKr—we reduce trainable parameters to 0.35\%, achieving high computational efficiency without sacrificing accuracy. Our model achieves over 90\% accuracy across tasks, demonstrating the robustness of this adaptive approach for real-time applications. This work provides a scalable solution for integrating scene contextual and pedestrian behavioral data in ADAS, enhancing pedestrian safety in autonomous systems.
- Juicer: Improving Latency in Geo-Replicated Databases
-
The goal of this project is to optimize latency in geo-replicated databases by developing and implementing a novel queue management and transaction reordering system. This project focuses on Juicer, a latency-optimization layer within the CockroachDB key-value layer. The key innovation in this project is the use of intelligent queue management and transaction reordering strategies, specifically designed for CockroachDB's distributed architecture. By addressing the challenges inherent in handling concurrent transactions across geographically distributed nodes, Juicer aims to reduce transaction wait times and improve response times without using machine learning, relying instead on heuristic-based queue management.
- Collaborative Sensing
-
This project employs collaborative sensing technology to optimize communication among vehicles, effectively addressing blind spot issues. By sharing environmental data, vehicles enhance traffic safety and operational efficiency.
- SLAM in Space
-
Achieving vehicle autonomy in space presents unique challenges due to the complexities of extraterrestrial environments. Simultaneous Localization and Mapping (SLAM) is a key technique enabling vehicles to navigate and map unknown terrains autonomously. This project explored terrestrial SLAM methods applied to various space-like environments, highlighting the limitations and obstacles faced in achieving reliable space autonomy. The findings provide valuable insights into advancing autonomous navigation technologies for future space exploration.
- RC Car with HID-Based Control
-
This project involves the design and implementation of a remote-controlled car, which can be driven using USB HID devices. The system utilizes an ARM-based microcontroller and various embedded systems protocols for user input and motion control.
- InsightBot
-
InsightBot is a dynamic Retrieval Augmented Generation (RAG) application that transforms how users interact with diverse content. Users can upload YouTube links, documents, and text files to create a rich, queryable knowledge base. The system processes and indexes content in real-time, using advanced AI to understand user queries and retrieve relevant information. As content is added or modified, InsightBot automatically updates its knowledge base, ensuring responses are always current. This versatile tool is ideal for research, content analysis, customer support, and knowledge management, offering a comprehensive solution for extracting valuable insights from various information sources.
- UB Media Forensic Lab
-
Deepfake o meter
- MLLM hateful video detection
-
This project aims to create a state-of-the-art multimodal hateful video detection system that integrates advanced machine learning techniques to address the challenges of hate speech in video content. Using the HatefulVideo dataset, the project evaluates various state-of-the-art vision models and employs comprehensive error analysis to identify gaps in existing methods. The model will leverage visual, audio, and transcript data, applying causal reasoning to detect relationships within videos and enhance understanding of complex contexts such as emerging slang and memes. Chain-of-thought methods will further strengthen the model's reasoning capabilities, enabling detection of nuanced and evolving hate speech trends.
- Spinning Rust
-
An on-disk Datalog engine for program analysis.
- HBML Lab
-
wSignGen: Word-Conditioned 3D American Sign Language Motion Generation
(CSE 4/555 Pattern Recognition)
- Multimodal Video Emotion Recognition in the wild
-
The Multimodal Video Emotion Recognition project focuses on developing a robust AI system that accurately detects and interprets emotions from diverse data streams, including video, audio, and facial expressions. Leveraging advances in deep learning and multimodal fusion techniques, this project integrates visual cues from facial expressions, auditory signals like tone and pitch, and contextual video content to recognize and classify emotional states more effectively than single-modal approaches. The model combines features from each modality, using specialized architectures such as CNNs for facial analysis, LSTMs or transformers for temporal sequences in video and audio, and multimodal attention mechanisms to weigh the importance of each input. By fusing these data streams, the project aims to enhance emotion recognition accuracy and provide insights into real-time applications, such as improving human-computer interactions, video content analysis, and mental health monitoring.
- Predicting the Podium: F1
-
This project delves into the world of Formula 1 racing, leveraging historical race data and statistical techniques to predict future race outcomes and driver performance.
- Protein Structure Prediction
-
Predict and compare the effectiveness of Hidden Markov Models (HMMs) and Convolutional Neural Networks (CNNs) separately in predicting the secondary structure of proteins, analyzing how each model handles sequence dependencies and spatial patterns within amino acid sequences.
- QBSNet: NFL Quarterback-based Analytical Prediction on an Offense's success per play
-
A Machine Learning project based around predicting an NFL offense's play outcome based off of the Quarterback's actions.
- Dynamic Facial Expression Analysis for Video/ Photo Based Emotion Recognition
-
LSTM based model to detect the changes in emotions and facial expressions from input video or pictorial data
- Using diffusion model to impute spacialtemporal data
-
Using diffusion model to impute spacialtemporal data
- Zxora: Multimodal Mental Health Chatbot
-
A uniquely empathetic AI companion that reflects not just your words, but your emotional state. Built on lean, mean, quantized open source models, Zxora is an emotional chameleon that learns to speak in your voice while reading between the lines of your texts. It’s a virtual confidant that can blend empathy with information retrieval from your own datasets and image generation from your text.
(CSE 4/546 Reinforcement Learning)
- AutoDrive-MARL: Autonomous Driving Multi-Agent Reinforcement Learning Optimization
-
This project aims to develop an efficient multi-agent reinforcement learning algorithm applied to autonomous driving environments. Based on the A3C architecture, we implemented both the A3C algorithm and an enhanced version of the PPO algorithm. By introducing entropy-based exploration strategies, we enhanced the robustness of the PPO algorithm, and by employing memory optimization techniques, we reduced resource consumption. To improve the parallel efficiency of the algorithm, we parallelized it using multiprocessing. During parameter updates, we utilized resource locks and checking mechanisms to ensure safe and efficient inter-process synchronization. The optimized algorithm achieved near-global optimal performance in the TrafficJunction10-v0 simulation environment.
(CSE 611: Master's Capstone Project)
- Niagara Aerospace Museum Scavenger Hunt
-
An interactive scavenger hunt for the Niagara Aerospace Museum. A mobile web application prompts players with questions to find different exhibits within the museum. Once an exhibit is located, scanning its NFC token reveals if it’s the correct match. Players or teams compete for the fastest completion times and earn themselves a spot on the leaderboard.
- Look The Part
-
Look The Part is an innovative mobile application that combines fashion technology and personalized styling to help users look and feel their best. The app provides tailored outfit recommendations making fashion accessible, sustainable, and effortless.
- Maverick Health
-
Maverick Health is a comprehensive health tracking app designed to empower users to take charge of their well-being through personalized assessments and actionable insights. The app offers a dynamic approach to health management by integrating multiple features that help individuals monitor, learn, and improve their overall health.
- RedPrint
-
- Revanth Balineni
- Vamsi Krishna Bellam
- Dhanush Babu Ramadoss
- Kishore Nithin Sridhar
- Sumana Madhireddy
The RedPrint Project focuses on streamlining gym management processes, enhancing user experience, and providing efficient communication between trainers, staff, and gym members. The project explores user role management, user engagement, and real-time data tracking to improve gym operations.
- Request Tracker AI
-
In request tracker AI we have included the features such as sentiment analysis of the ticket, ticket summarisation of stream of tickets on a particular issue, text autocompletion to assist the users with suggestions to their content and text translation within multiple languages. This features will provide assistance to users and enhance their experience with the product.
- Safety Knights
-
Provide a virtual social space for EHS safety professionals to connect, network, and discuss about safety.
- Kaleida Health
-
Build a comprehensive system featuring Patient, Doctor, and Admin portals. The Patient portal should allow patients to view medical reports, daily status updates, assignments, and notifications. They should also be able to communicate with doctors through an integrated chat system, which includes a translation feature for multilingual support. The Doctor portal should enable doctors to securely access and manage patient data, update treatment plans, and monitor daily patient status, while also communicating with patients using the chat system with translation capabilities. The Admin portal should provide tools to oversee and administer organizational data, manage user roles (patients and doctors), and handle administrative tasks such as assigning roles and generating notifications. The system must ensure secure handling of all data and maintain robust privacy standards across all portals.
- Youro
-
- Amrutha Pullagummi
- Sai Savithru Mohan Pishka
- Tharun Gangaraju
- Vamsikrishna Jayaraman
- Madhuri Reddy Baradi
Youro is a health platform that connects patients with urologists for convenient virtual care, making urologic healthcare more accessible, straightforward, and personalized. We offer virtual consultations, 24/7 expert advice, and seamless coordination for video appointments to address a range of urologic concerns, including recurring UTIs, kidney stones, and erectile dysfunction. With a strong focus on privacy, evidence-based treatments, and patient-centered care, Youro combines advanced technology with skilled specialists to deliver high-quality, tailored solutions. Our personalized diagnosis questionnaire collects vital details about symptoms and medical history to ensure optimized diagnostics, while our symptom severity scores provide valuable insights for informed decisions. Patients receive customized care plans, including detailed notes, prescriptions, and feedback from doctors. Additionally, Youro promotes holistic wellness and proactive healthcare through its My Diagnosis section, empowering individuals with the knowledge to make informed health choices.
(CSE 546: Reinforcement Learning)
- Daring Dave: An RL Approach to Dangerous Dave
-
In this project, we explore the application of advanced reinforcement learning (RL) techniques to the classic platform game Dangerous Dave, a game renowned for its sparse rewards and challenging navigation. Drawing inspiration from the success of exploration-focused algorithms like Random Network Distillation (RND) and Go-Explore in similarly complex games, we adapted these approaches to improve learning efficiency and agent performance in this unique environment. By designing a custom game environment tailored to RL frameworks and iteratively refining the reward structures, we achieved significant advancements in exploration, learning efficiency, and the agent's ability to generalize strategies to new levels, offering valuable insights into overcoming challenges in sparse reward settings.
- Team "AInspire"
-
Advanced Deep Reinforcement Learning for Traffic Signal Optimization in Vehicular Networks Using SUMO and TraCI - This project leverages deep reinforcement learning (DRL) and real-time vehicular network data, simulated in SUMO, to optimize traffic signal control. Unlike traditional fixed-duration methods, our approach models intersections as grids, using advanced techniques like DQN, A2C, and PER to improve traffic flow, reduce delays, and enable adaptive traffic management.
- Project: Reinforced Learning for Real-Time Network Traffic Path Optimization
-
In this project, we are focusing on improving the real time network traffic using the Reinforcement Learning algorithm by dynamically optimizing the routing paths and exponential growth in data and devices connected over the networks. We have designed the environment for our proposed dynamic network routing problem and applied several Reinforcement Learning algorithm like Q – learning, DDQN, Actor-Critic to simulate our traffic network.
(CSE 442: Software Engineering)
- Sugarmen from Sugartown
-
A python based 2D local multiplayer fighter game
- GitGraph
-
GitGraph serves as a companion to GitHub. Users can view view information about their repositories in graph formats, search commit messages, sort through branches, and more in this interactive web app.
- TDB
-
TBD aims to be the premier music recommender, revolutionizing music discovery by seamlessly connecting listeners with tracks that fit their tastes while encouraging exploration of new genres and artists. Our vision is to create a deeply personal listening experience that evolves with each user, adapting to their changing moods and preferences over time. As listeners grow, TBD will grow with them, providing a dynamic and evolving music discovery journey.
- Stop Buggin
-
" Stop Buggin' " is a fun and engaging tower defense game where players use animal towers to protect against waves of invading bugs. Designed with young teenagers in mind, the game offers simple yet strategic gameplay with bright, cartoonish visuals and a focus on accessibility. Players will build and sell towers, earn food as currency, and progress through levels with increasing challenges. With a tutorial for new players and progressive difficulty increase for veterans, "Stop Buggin'" delivers an enjoyable and rewarding experience for all skill levels.
- C5-Note
-
A powerful note-taking and organization app designed for seamless real-time collaboration and effortless productivity. Group and organize content dynamically within pages, enabling clear structure and easy navigation. With live editing and sharing, teams can stay connected and aligned, transforming ideas into action.
- Survival Island
-
A survival game where you gather resources, develop a base, and fight enemies with the goal of finding a way off of an island
- DormRaters
-
DormRaters is an online platform where students can find and review local dorms, helping others make informed choices. Our Mission At DormRaters, we believe in the power of local communities. Our mission is to connect people with great dorms, fostering a space where consumers can share honest feedback and discover trusted recommendations. From local to graduate students, we’re here to elevate voices, celebrate authenticity, and support the vibrant tapestry of campus life. By connecting consumers and businesses, we aim to build stronger communities and create meaningful connections that empower every user to make informed choices.
- CS Clowns
-
Are you a student or a person who takes a lot of notes? Do you struggle to find them in an endless abyss with no dates, subjects, or titles? Have you ever missed a lecture and needed your buddy to share their notes? Well, look no further than our web app called Noted! This collaborative web app allows users to take, organize, and share class notes using "Course pools". In addition, our app has the following features listed below: Notes Annotation! We know plain simple text can be difficult to study so we added the ability to highlight and draw on your notes so that the most important concepts can be emphasized! Live Chat is akin to Discord. Users can chat with one another via chat messages To-Do and Reminders Section where users can make a Do list, set a reminder, and have it appear in their calendar Custom Profile Making! Users can add images, and create a Bio! A sense of belonging is fostered for those who long to be in a community that evokes educational success and growth. Whatever you need we got that Noted! 👊
(CSE 446)
(CSE 460/560)
- The Science of Scoring: Evaluating Efficiency Among Europe’s Top Strikers
-
This project aims to analyze the efficiency of football/soccer strikers across Europe’s top leagues using data from 2022/2023. Our targeted performance metrics, including goals scored, assists contributed, dribbles made etc, are processed and analyzed using PySpark’s distributed capabilities for scalable data cleaning and machine learning. The insights help identify top-performing strikers, offering valuable data-driven guidance for player recruitment and performance management.
(CSE 487)
- DiffReg: Diffusion Model for Point Cloud Registration
-
Our Project we are presenting is DiffReg, a novel diffusion-based method for 3D point cloud registration. Inspired by diffusion models, DiffReg iteratively refines the predicted correspondences between two point clouds by progressively denoising their coordinates. This approach achieves state-of-the-art performance on both ModelNet and 3DMatch.
(CSE 587: Data Intensive Computing)
- Neural Nomads
-
Network Attack Detection using Machine Learning
(CSE 546 - Reinforcement Learning)
- Reinforcement Learning powered : Movie Recommendation Engine
-
We have Built a movie recommendation engine using Reinforcement Learning Algorithms ( DDPG) that leverages user and movie embeddings, genre preferences, and reinforcement learning to personalize recommendations dynamically. Traditional recommendation systems rely on static collaborative filtering or content-based techniques. Incorporating reinforcement learning improves adaptability and decision-making by actively optimizing recommendations for user satisfaction.